The Future of Pharmacovigilance and AI
March 31st 2023
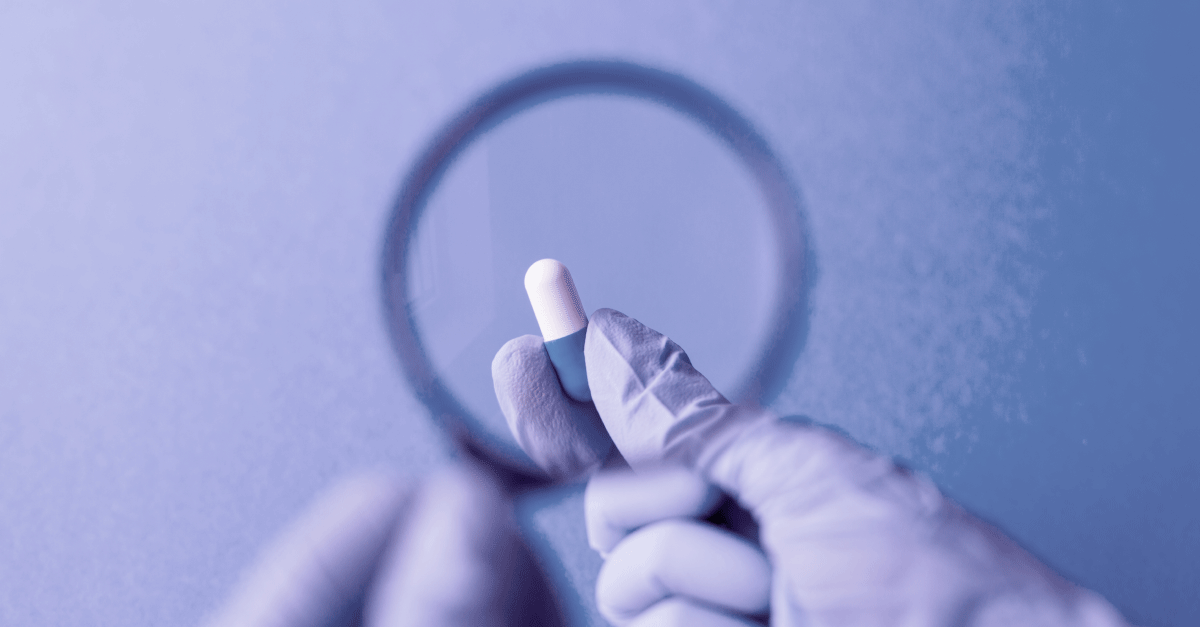
With the increasing number of drugs being introduced in the market and the complexity of their effects on human physiology, ensuring drug safety has become more challenging than ever. Keep reading to find out how the pharmacovigilance industry is making the most of AI with new trends.
The use of artificial intelligence in pharmacovigilance has been steadily increasing over the past few years, and it’s poised to transform the industry in the years to come. AI-powered pharmacovigilance tools offer pharmaceutical companies, contract research organizations, and regulatory bodies the ability to detect safety signals earlier, improve the accuracy and completeness of adverse event reporting, and reduce the time and costs associated with pharmacovigilance activities.
As we look to the future of AI in pharmacovigilance, several key trends are emerging. In this post, we’re going to explore what these trends are and how they’re helping solve key challenges.
Key Trends
Increased use of NLP
NLP is a field of study that focuses on the interaction between computers and human languages. In pharmacovigilance, NLP is used to extract meaningful information from unstructured data sources such as social media posts, patient forums, and medical records. By using NLP, pharmacovigilance professionals can quickly identify potential adverse events associated with drugs and take appropriate actions to ensure patient safety.
Integration
Another trend is the integration of AI-powered pharmacovigilance tools with electronic health records (EHRs) and other healthcare data sources. This integration enables real-time monitoring of patient safety and can provide insights into drug efficacy and adverse events that were previously difficult to identify. In addition, the use of AI-powered pharmacovigilance tools is expected to improve the efficiency and accuracy of regulatory reporting. By automating the reporting process, AI can reduce the risk of errors and ensure that all relevant information is included in regulatory submissions.
Predictive analytics
One of the most promising areas of AI in pharmacovigilance is the use of predictive analytics. Predictive analytics involves the use of statistical algorithms to determine patterns and make predictions about future outcomes. In pharmacovigilance, predictive analytics can help identify safety signals before they become significant problems. By identifying potential adverse events early on, pharmacovigilance professionals can take appropriate actions to ensure patient safety and reduce the risk of harm associated with drugs.
Machine Learning
ML is a subset of AI that involves the use of algorithms to learn from data and make predictions or decisions without being explicitly programmed. In pharmacovigilance, ML algorithms can be trained on large datasets of adverse events to identify patterns and signals that may not have been detected using traditional pharmacovigilance methods. By using ML, pharmacovigilance professionals can improve the accuracy and completeness of adverse event reporting, leading to better patient outcomes.
Electronic Health Records (EHRs)
EHRs are digital versions of patients’ medical records that can be accessed and shared by healthcare providers. In pharmacovigilance, the integration of AI-powered pharmacovigilance tools with EHRs and other healthcare data sources enables real-time monitoring of patient safety. This integration can provide insights into drug efficacy and adverse events that were previously difficult to identify, allowing for more effective risk management.
Data Quality
Ensuring the quality and completeness of data used in AI-powered pharmacovigilance tools is critical to the success of pharmacovigilance. Poor quality data can lead to inaccurate predictions and decisions, potentially harming patients. Collaborative efforts between pharmaceutical companies, CROs, and regulatory bodies are necessary to ensure that data is accurate, consistent, and relevant.
Transparency and Accountability
The need for transparency and accountability in AI-powered pharmacovigilance cannot be overstated. Patients and healthcare providers must understand how AI algorithms are making decisions and how their data is being used. Clear explanations of how AI algorithms are making decisions and how data is being used can help build patient and healthcare provider trust in the pharmacovigilance process.
Digital Therapeutics
Digital therapeutics are a new class of therapeutic interventions that use software to treat or prevent medical conditions. In pharmacovigilance, digital therapeutics can be used to enhance drug safety monitoring and improve patient outcomes. By providing patients with digital therapeutics, healthcare providers can monitor patients’ health and detect adverse events associated with drugs in real-time.
Social Media
Social media is increasingly becoming an important source of pharmacovigilance data. Patients and healthcare providers use social media platforms to report adverse reactions and share their experiences with drugs. The use of social media in pharmacovigilance can enable the detection of safety signals that may not be captured by traditional methods.
Social media monitoring is becoming an increasingly popular method for detecting potential safety issues associated with drugs. By monitoring social media posts, pharmaceutical companies and regulatory bodies can quickly detect and respond to potential safety issues, potentially saving lives.
Blockchain Technology
Blockchain technology is a distributed ledger technology that enables secure and transparent sharing of data across stakeholders. In pharmacovigilance, blockchain technology can be used to ensure the integrity of data and enhance collaboration between pharmaceutical companies, CROs, and regulatory bodies.
Real-World Data
Real-world data refers to data collected outside of clinical trials, such as data from electronic health records, claims databases, and patient-generated data. In pharmacovigilance, the use of real-world data allows for the monitoring of drug safety and efficacy in real-world settings. This monitoring can help identify potential adverse events associated with drugs and improve patient outcomes
Conclusion
With the increasing number of medications and adverse reactions, traditional methods of pharmacovigilance are becoming less effective. However, with the advent of new technologies, such as predictive analytics, AI, and machine learning, the landscape of pharmacovigilance is changing rapidly.
Pharmaceutical companies need to invest in new technologies and methods and ensure compliance with pharmacovigilance regulations and guidelines to ensure drug safety and maintain regulatory compliance. The future of pharmacovigilance is bright, and the use of new technologies will revolutionize drug safety, leading to better health outcomes for patients.
Discover how our semantic AI platform and smart data capture and analysis system can strengthen your pharmacovigilance processes, optimize your practices, and empower your decision-making.
Ready to see what we can do for you?
In the right hands, artificial intelligence can take human performance to a hitherto unimaginable level. Are you ready for evolution?