Harnessing the Power of AI in Pre-Clinical Research
January 19th 2023
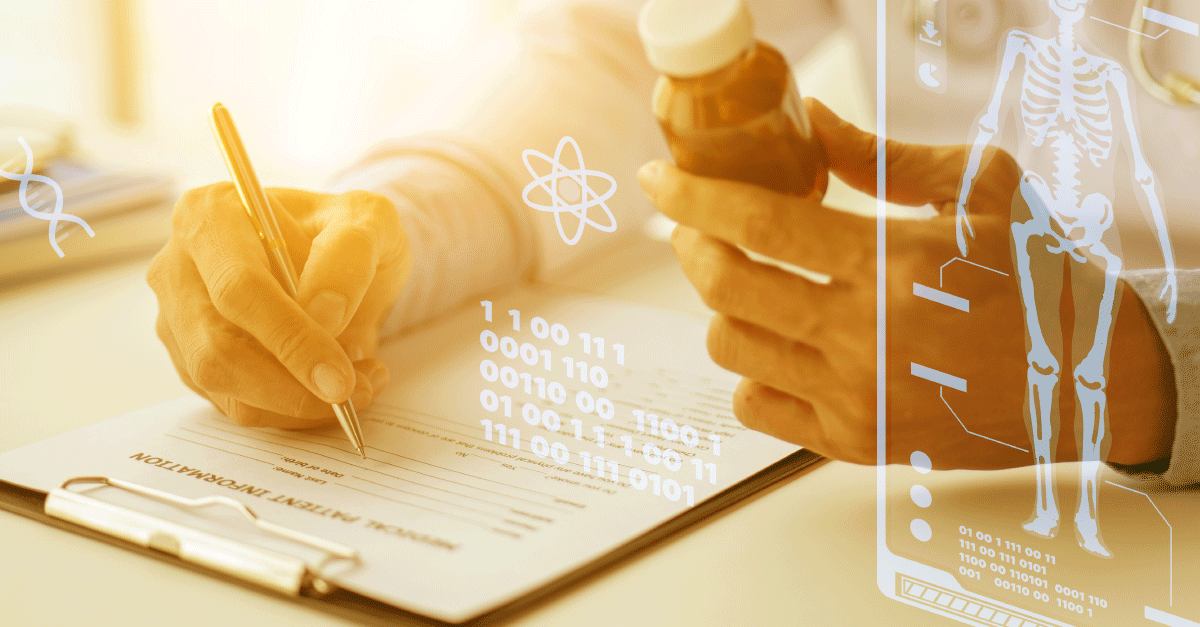
The use of AI in pre-clinical research is proving to be significantly powerful in supporting trial efficiency. AI automation has the potential to make previously time-consuming processes faster and more accurate, allowing for enhancing insights and better decision-making when it comes to clinical research. Let’s find out more.
Pre-clinical studies are an important part of the drug development process, as they help to evaluate the safety and efficacy of potential new drugs. The use of artificial intelligence can potentially enhance these studies by providing more accurate and detailed data.
When understanding the use of AI in pre-clinical studies, it’s important to consider the potential benefits and limitations of the technology. AI can help to identify patterns and trends in data that may not be obvious to human researchers, allowing for more accurate predictions and conclusions. It’s also essential to ensure that AI algorithms are properly validated and the data used to train them is accurate and representative.
Ethical concerns should also be acknowledged, including the potential for bias and the consequences of relying too heavily on AI in decision-making. Overall, the use of AI in pre-clinical studies can be beneficial, but it should be carefully evaluated and carefully integrated into the overall research process.
The Drug Discovery Stage
During the drug discovery stage, drugs are assessed and tested through pre-clinical research. At this point, the drug concept advances to the biological testing stage (in vivo and/or in vitro). Preclinical studies involve testing a drug, a diagnostic procedure, or other medical treatment on animals before trials can be carried out on humans. Testing new drugs on animals is a costly and time-consuming process, but it’s carried out to ensure their safety.
The 3R framework (Reduce, Refine, Replace) helps reduce animal testing by focusing on in vivo (animal model) testing. Alternatives to animal testing are necessary to study how new drugs affect human diseases.
The goal of preclinical research or trial is to generate enough data to determine that the drug is safe to proceed with human trials. This means that a CRO needs to provide data to the regulator to get approval for the next phase of drug development. The data contains information on efficacy and safety and is obtained by performing pharmacology and toxicology studies. Pharmacology studies are concerned with the pharmacokinetics and pharmacodynamics of a drug, toxicology deals with toxicity, including genotoxicity.
Challenges
One major challenge for a pre-clinical study during drug development is obtaining reliable and reproducible results. This is due to the complex nature of the study, which involves testing the drug on animal models and observing its effects on various biological processes. There might be variations in the responses of different animal models, making it difficult to accurately predict the drug’s effects on humans.
Another challenge is ensuring the safety and ethical use of animal subjects in the study. Here the main issues are cost and time. Huge amounts of scientific data need to be collected and analyzed, be it text or images. Potential test recipients need to be discovered in a meaningful way too. Also, numerous animal models and tissue samples need to be evaluated. It involves scientific research and understanding the regulatory context of each step, including safety, dosage, efficacy, etc.
It’s no surprise that this part of the drug development process is not only outsourced to contract research organizations but also implicates artificial intelligence tasks, such as automation and discovering relationships.
The Role of AI & Contract Research Organizations (CROs)
Looking at the current situation it’s clear that if a CROs’ research output lacks speed, accuracy, cost reduction, and above all meaningful results, then the existing high failure rate will continue to increase. This will result in even higher stranded costs and most importantly, the failure to discover new drugs. AI and its predictive capabilities help to improve amortization. However, AI can only complement the humans involved in the process and provide support for decision-making.
AI has a significant impact on data analysis during pre-clinical studies. When used by CROS, AI can help identify patterns and trends in data faster and more precisely than manual analysis. Without the use of AI, CROs may face challenges in accurately and efficiently analyzing data, potentially delaying the progress of the study and affecting the overall success of the drug development process.
Benefits of Using AI in Pre-Clinical Research
The payoff for a contract research organization (CRO) if it makes use of AI during a pre-clinical study could be significant. Some potential benefits of using AI in this context may include:
1. Increased efficiency and productivity
There are lots of tasks that AI algorithms can automate to improve business efficiency and productivity. One of these is data analysis, which can speed up the pre-clinical study process and allow CROs to handle more studies in a shorter amount of time.
2. Improved accuracy and precision
AI algorithms can be trained to identify patterns and trends in data that may not be obvious to human analysts. This can lead to acquiring more accurate and precise results from the pre-clinical study.
3. Better decision-making
CROs can benefit greatly from the insight and recommendations that AI provides based on the data collected during the pre-clinical study. This analysis will lead to informed decisions about the study’s design and results.
4. Enhanced data management and analysis
By using AI algorithms to manage, organize, and analyze large amounts of data generated during a pre-clinical study, it is easier for CROs to monitor the study’s progress and identify potential issues.
Conclusion
AI is a powerful tool for pre-clinical studies, enabling automation of data collection, analysis, and simulation; identification and selection of animal models; and monitoring and control of study conditions. The capacity AI has to identify patterns and trends, predict outcomes, and optimize the design and accuracy of studies, is an invaluable resource for pre-clinical research.
Another key aspect involved in the drug development process is medical writing. This is required to communicate both with the research community as well as with the regulators. AI is an excellent tool that is advantageous to medical writers and supports quality control during the drug development process. This involves providing numerical data directly to the writer using databases. Also, semantic search and summarization, and support for a lean medical writing style. Look out for more about this in one of our future posts
Head over to our blog to check out more about the drug development process.
Ready to see what we can do for you?
In the right hands, artificial intelligence can take human performance to a hitherto unimaginable level. Are you ready for evolution?