CROs, Artificial Intelligence, and the Stages of Drug Development
December 20th 2022
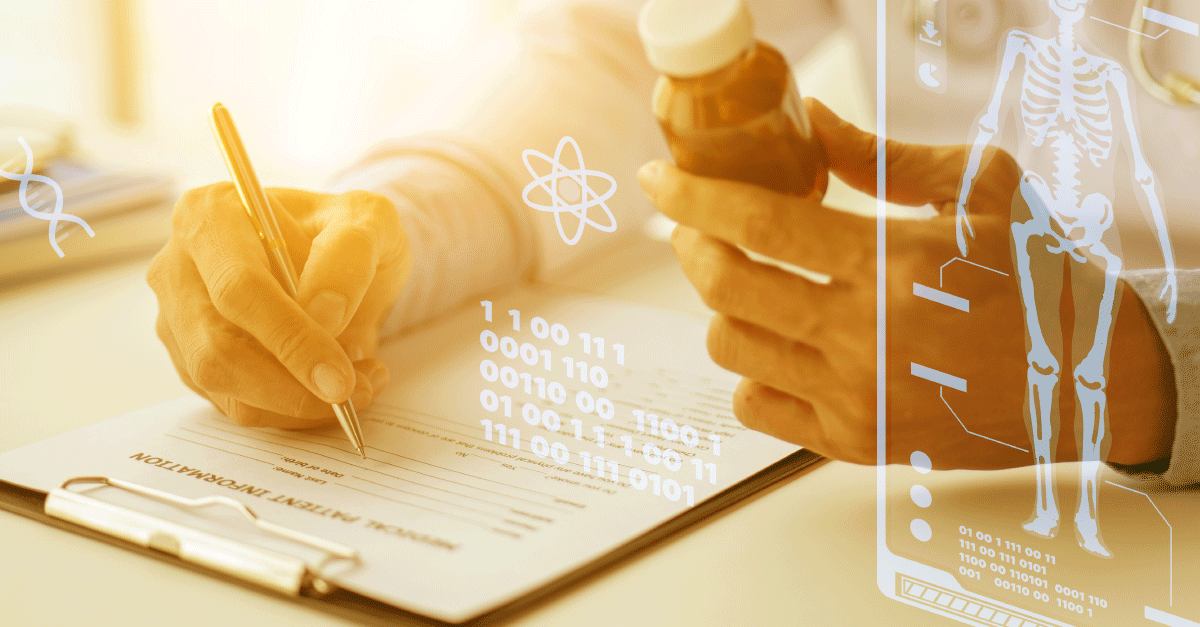
Contract Research Organizations (CROs) need to apply a proactive approach and introduce AI-based methods and strategies to ensure future success. AI plays a significant role in drug development, from the research and discovery phase to launching a new drug to market. We’re going to take a closer look at the stages of drug development and highlight potential challenges and solutions.
Technology and consumerism have been significant driving forces for society in recent years. This means that Contract Research Organizations (CROs) that can provide state-of-the-art products and services to trial sponsors will have an advantage. Companies can keep their competitive edge by innovating and providing the best possible products and services.
CROs have long been responsible for following protocols specified by clinical trial sponsors. However, this approach isn’t enough to ensure sustainable success in the future. Artificial intelligence will play a central role throughout the entire drug development lifecycle. To maintain uninterrupted progress, CROs must take a more proactive approach that considers the unique needs of clinical trial sponsors. At the same time, they’ll need to introduce AI-powered methods and strategies. By doing so, CROs can establish successful and beneficial trials for the patients involved.
How Can We Involve AI?
AI has become a key player across healthcare, life science, and pharmacovigilance. When it comes to conventional drug discovery takes between 10 to 15 years and costs billions of dollars. Despite the costs associated with failures, accounting for 75% of total R&D costs, these setbacks nonetheless contribute to our understanding of the disease process. This knowledge is invaluable in the fight against diseases. Leveraging this data and turning it into actionable insights is what AI will deliver to CROs, hence the clinical trial sponsor. Or as Deep Pharma summarized it (Artificial Intelligence for Drug Discovery Landscape Overview Q1 2022).
The solution to this problem comes from 3 key strategies:
- Evolution of business models towards more collaboration and early pipeline diversification
- Implementation of AI as a universal shift toward data-centric drug discovery
- Discovery of new therapeutic modalities (biologics, therapies, etc.)
The implementation of AI in the drug development process
Let’s take a look at the second strategy in more detail: the implementation of AI in the drug development process.
The drug development process is based on understanding huge amounts of data. Data scientists rely on this information to drive scientific progress, but big data presents unique challenges. Researchers often lack access to information beyond professional articles, patents, patient data, and other unstructured data that reference their field. As a result, they miss important findings that could support or contradict their work.
AI can effectively explore large amounts of data to reconcile and match data constantly. Researchers can use AI to help identify targets for drug discovery, design new drugs, and repurpose old drugs.
We have observed a lot of improvements over the last 5 years regarding AI, particularly its application during drug development. The upcoming 2 years will likely shape the future of CROs and trial sponsors, depending on how they implement AI into the value chain.
The Drug Development Stages
There are 8 phases a successful drug will need to pass to be successfully marketed (Koshechkin, K.A.; Lebedev, G.S.; Fartushnyi, E.N.; Orlov, Y.L). We’re going to focus on the early research and development phase.
The goal of R&D is the development of new molecules and the identification of new biological targets. The lifecycle of a pharmaceutical product begins with rigorous research and development. The first stage involves searching for the composition of a medicinal product, most often molecules, and determining its biological activity based on the properties of its components. It also includes the identification of drug targets; these are molecular structures whose activity is associated with a disease. This leads to data analysis of patient samples, prediction of pharmacological properties, and the simplification of protein design. An additional focus of research ventures into new technologies of future production.
The discovery phase is usually one of the most expensive and time-consuming when launching a new drug to market. It can take up to 5 or 6 years. The implication is that even though it costs more to develop new drugs fewer are brought to market. The traditional approach to drug design involves laborious manual processes in which the researcher conducts a series of experiments. AI can improve and expedite this process and not only point the way to new drugs but also reduce the cost and time for this phase.
Applying AI to this phase involves screening drug compounds and determining proper biological targets. The sources for this work are structured and most often unstructured scientific papers and data repositories that deals with genes, drugs, diseases, and more biological and chemical entities. It’s an ever-growing treasure trove, that is too large for humans to process without assistance. Searching for new drugs based on machine learning leads to a decrease in drug combinations that are significantly different from existing methods and a decrease in the diversity of candidate substances.
AI & ML Tools
Around 50% of AI use cases in drug development concern the discovery of novel molecules or target identification. Natural Language Processing (NLP) can drastically improve the analysis of a diverse range of content and support decision-making with the help of smart information retrieval practices. The objective is to uncover hidden relations in large data sets. It’s similar to deconstructing these datasets and revealing new structures and relationships. These tasks can be achieved with a diverse set of AI and ML tools.
1. Convolutional neural networks (CNNs):
CNNs are well-suited to analyzing and interpreting images, such as medical images. They can be used to identify patterns and features in images, which can help to inform the drug development process.
2. Recurrent neural networks (RNNs)
RNNs analyze and interpret sequential data, such as genetic sequences or electronic health records. They can be used to identify patterns and relationships in this data, which can help to inform the drug development process.
3. Generative adversarial networks (GANs)
GANs can be used to generate new data, such as virtual 3D models of potential drug molecules. They can be trained on large datasets of existing drug molecules and can then be used to generate new molecules that can be tested and optimized using other deep learning algorithms.
4. Reinforcement learning (RL)
RL can be used to optimize the design of new drug molecules. These algorithms can be trained on datasets of known drugs and their effects and can then be used to make predictions about the potential effects of new drug candidates. This can help to prioritize which candidates should be further tested.
Further approaches include Deconvolutional Neural Networks (DNN) or pre-trained Transformers, dictionary-based analytics, statistically supervised learning models such as Long short-term memory networks (LSTM), Support Vector Machines (SVM), and Random Forests (RF), to name a few.
Conclusion
When it comes to providing AI applications for drug development there are certain areas where you need to shine, these include:
- Expertise in deep learning algorithms and techniques, including the ability to design, implement, and evaluate deep learning models for a variety of tasks and applications in drug development.
- Strong programming skills, particularly in languages and frameworks commonly used for deep learning, such as Python and TensorFlow.
- Familiarity with tools and platforms commonly used for data storage, processing, and analysis, such as cloud computing platforms, databases, and data management tools.
- Knowledge of best practices and principles for developing and deploying AI applications, including design patterns, testing and validation techniques, and security and privacy considerations.
- Experience working with large and complex datasets, such as genetic sequences, medical images, and electronic health records, and the ability to clean, pre-process, and analyze these datasets using deep learning algorithms.
- Familiarity with visualization and reporting tools, such as dashboards and data visualization libraries, to present results and insights from the AI application in a clear and effective manner.
Take a look at the LifeScience solutions we can offer to improve decision-making.
Ready to see what we can do for you?
In the right hands, artificial intelligence can take human performance to a hitherto unimaginable level. Are you ready for evolution?