The Battle of Innovations: Generative AI vs Predictive AI Explained
9th May 2024
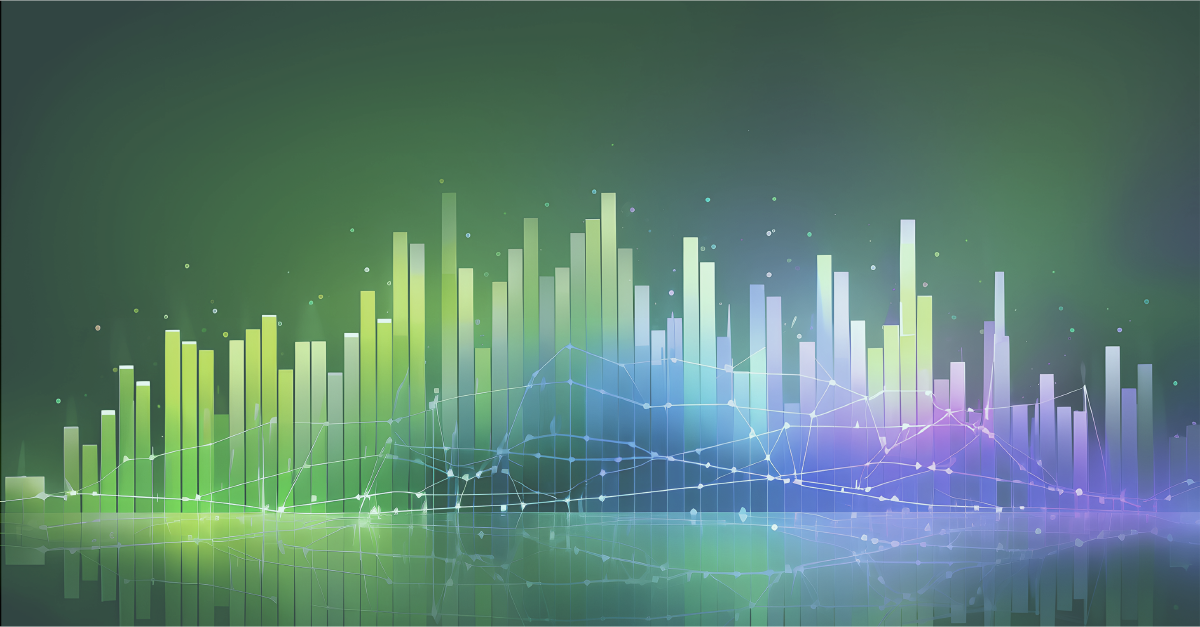
The battle between Generative AI and Predictive AI is reshaping industries, but which powerhouse will fuel your next breakthrough?
Generative AI crafts novel content and designs, pushing creative boundaries, while Predictive AI analyzes trends and data, forecasting the future with uncanny accuracy. As a business leader, your choice between these technologies could either unlock a treasure trove of opportunities or leave you scrambling in the wake of more agile competitors.
The tech world is buzzing with excitement over generative AI definition – a cutting-edge approach to artificial intelligence that can generate human-like text, images, and more from scratch. While predictive AI techniques like machine learning focus on analyzing data to make predictions, generative AI models like text generation use deep learning to create entirely new content.
Each holds the key to not just surviving but thriving in a future that values foresight and creativity. Imagine harnessing Generative AI to bring unprecedented creativity to your products or employing Predictive AI to anticipate market shifts with precision.
This isn’t just about keeping up; it’s about leading with confidence and insight. Are you ready to uncover which AI will be your ally in carving out a future where your business doesn’t just grow, it leads? In today’s blog post, we’ll help you decide which will be the cornerstone of your strategic arsenal.
Generative AI Explained
The Creative Force Behind Generative AI
Generative AI is switching up the way we create and consume content. At its core, it focuses on generating new and original data, such as text, images, audio, and video, by learning from existing patterns in vast datasets. Unlike predictive AI, which forecasts future outcomes based on historical data, generative AI unleashes a world of possibilities by crafting entirely novel creations.
How Does Generative AI Work?
- Data Ingestion: Generative AI models ingest massive amounts of data, such as text, images, or audio files, to learn the underlying patterns and relationships.
- Training: Using advanced deep learning techniques like neural networks, generative adversarial networks (GANs), and transformers, the model is trained to understand and replicate the patterns found in the training data.
- Generation: When provided with an initial input, such as a text prompt or an image seed, the generative AI model can generate new, original content that mimics the learned patterns while introducing novel elements.
What Are The Main Capabilities and Applications of Generative AI?
Generative AI’s ability to create new content from scratch opens up a wide range of applications across various industries:
- Content Creation: Automating the generation of articles, stories, scripts, and even code, streamlining creative workflows and reducing manual effort.
- Design and Art: Generating unique designs, images, and artwork, empowering artists and designers with new creative tools.
- Synthetic Data Generation: Creating realistic synthetic data for training other AI models, particularly in domains where real-world data is scarce or difficult to obtain.
- Personalized Experiences: Tailoring content, recommendations, and experiences to individual preferences and contexts, enhancing user engagement and satisfaction.
- Language Translation and Localization: Generating high-quality translations and localized content, bridging language barriers and enabling global communication.
The Most Important Advantages and Limitations
While generative AI offers numerous benefits, it’s essential to acknowledge its limitations and potential risks:
Advantages:
- Automation of creative tasks and content generation
- Ability to work with unstructured data
- Diverse and novel output
- Scalability and efficiency
Limitations:
- Potential for generating biased or inappropriate content
- Hallucinations and factual inaccuracies
- Resource-intensive training and high computational costs
- Ethical concerns around data privacy and intellectual property
Predictive AI Explained
The Power of Foresight
While generative AI captivates us with its ability to create, predictive AI harnesses the power of data to forecast the future. This branch of artificial intelligence leverages machine learning algorithms and historical data to identify patterns and make predictions about upcoming events, trends, and outcomes.
How Does Predictive AI Work?
- Data Ingestion: Predictive AI models ingest vast amounts of structured and time-series data from various sources, such as sales records, customer interactions, and sensor readings.
- Pattern Recognition: Using advanced machine learning techniques, the model analyzes the data to identify patterns and relationships that can be used to make predictions.
- Model Training: The predictive model is trained on historical data, learning to recognize patterns that have led to specific outcomes in the past.
- Prediction: When new data is fed into the trained model, it can make predictions about future events or outcomes based on the identified patterns.
What Are The Main Applications and Benefits of Predictive AI?
Predictive AI’s ability to provide actionable insights and aid in decision-making has made it invaluable across industries:
Industry | Applications |
Retail | Demand forecasting, inventory optimization, customer targeting |
Finance | Risk assessment, fraud detection, financial forecasting |
Healthcare | Disease outbreak prediction, preventive care |
Manufacturing | Predictive maintenance, supply chain optimization |
Marketing | Targeted campaigns, churn prevention, personalized experiences |
The benefits of predictive AI include:
- Automating complex analytics and simplifying data processing
- Improving accuracy and success rates of business decisions
- Extracting more value from data by providing forward-looking insights
- Streamlining operations and optimizing resource allocation
What Do You Have To Consider With Predictive AI?
While predictive AI offers numerous advantages, it’s essential to be aware of its limitations:
- Reliance on the quality and availability of historical data
- Potential for amplifying biases present in the underlying data
- Difficulty interpreting complex predictive models (lack of interpretability)
- Resource-intensive training and deployment processes
- Ethical concerns around privacy, bias, and discrimination
Generative AI vs. Predictive AI: The Key Differences
Objective and Function
Generative AI models are designed to generate new, original content or data from scratch, such as text, images, audio, or video. In contrast, predictive AI models analyze existing data patterns to make informed predictions or forecasts about future outcomes.
Training Data
Generative AI requires diverse and comprehensive datasets to learn the underlying patterns and relationships necessary for generating novel content. On the other hand, predictive AI relies heavily on historical data to identify patterns and make predictions based on past events or trends.
Learning Process
Generative AI models learn by ingesting vast amounts of data and using advanced techniques like neural networks and transformers to understand and replicate the patterns found in the training data. Predictive AI models, on the other hand, learn from historical data using machine learning algorithms to recognize patterns that have led to specific outcomes in the past.
Applications and Use Cases
Generative AI excels in creative tasks and content creation, such as generating articles, stories, designs, artwork, and even code. Its ability to produce novel outputs makes it invaluable in industries like media, advertising, and entertainment.
Predictive AI, on the other hand, shines in areas like business analytics, financial forecasting, risk assessment, and supply chain optimization. Its strength lies in providing data-driven insights and predictions that can inform decision-making and optimize processes.
Complexity and Resource Requirements
Aspect | Generative AI | Predictive AI |
Training Complexity | Generally more complex and resource-intensive | Less complex training compared to generative models |
Model Size | Large language models with millions of parameters | Relatively smaller models with fewer parameters |
Computational Resources | Requires significant computational power and resources | Less resource-intensive than generative AI |
Creativity and Novelty
One of the key advantages of generative AI is its ability to create entirely new and novel content, unleashing a world of creative possibilities. Predictive AI, on the other hand, is limited to making predictions based on existing patterns and historical data, lacking the element of content creation.
Final Takeaways
The advancements in generative AI and predictive AI are opening up new frontiers in numerous industries, from healthcare and manufacturing to media and entertainment. While generative AI excels in creating novel content and unlocking new realms of creativity, predictive AI provides invaluable insights and forecasts to optimize processes and inform data-driven decision-making. As these technologies continue to evolve, it’s essential to navigate their ethical implications proactively, addressing concerns around bias, privacy, intellectual property, and workforce displacement.
Find out how our Virtual Agent can catapult your business into the future.
Don’t miss out on our weekly blog posts and LinkedIn updates about the fascinating advancements that will propel your business.
Ready to see what we can do for you?
In the right hands, artificial intelligence can take human performance to a hitherto unimaginable level. Are you ready for evolution?